Fire Detection
In the era of climate change and increasing wildfire events, the ability to detect fires early and accurately is more critical than ever. Fires pose significant threats to ecosystems, agriculture, infrastructure, and human life. As part of our Satellite-as-a-Service platform, we have extended our AI capabilities by developing a high-performance, on-board fire detection system, optimized for embedded hardware and designed to run directly on edge devices without requiring internet connectivity or cloud infrastructure.
A sneak pic to what we can do
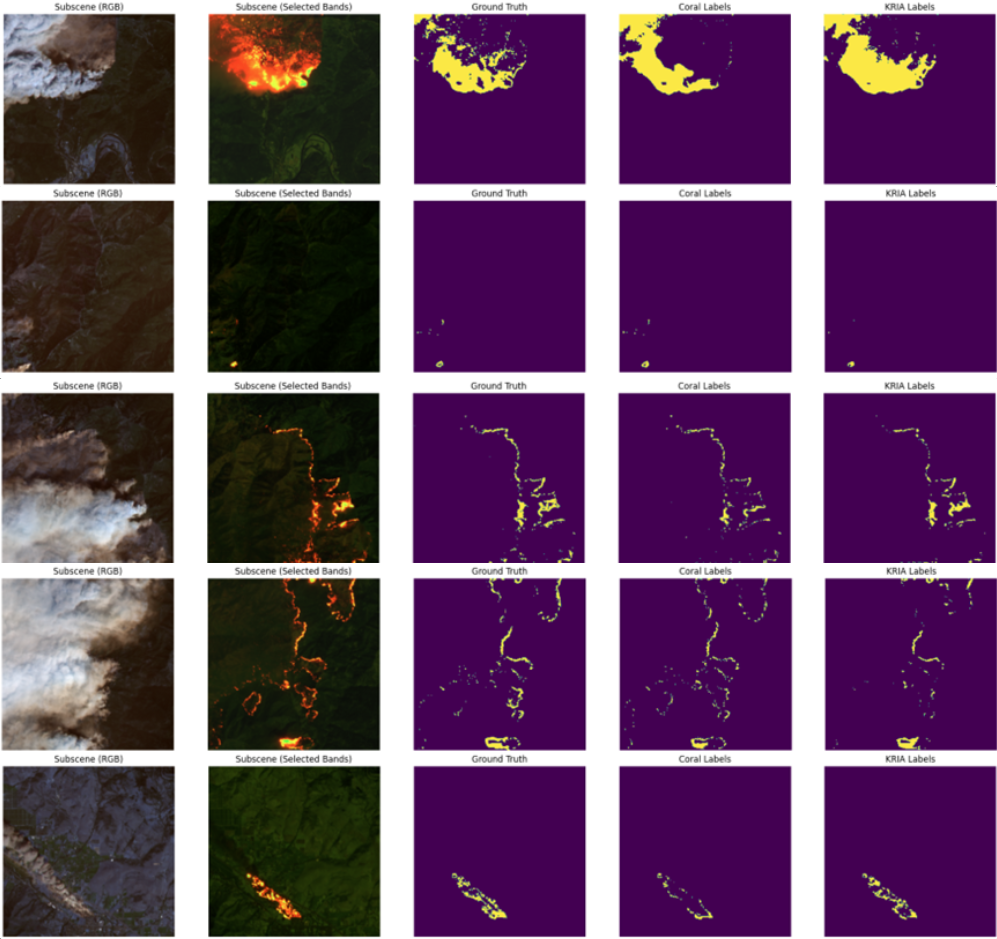
The model is optimized for lightweight inference on embedded platforms like Google Coral and Xilinx KRIA, enabling real-time processing without cloud dependency. Designed for drones, satellites, and monitoring stations, it ensures rapid decision-making even in remote areas. It generates interpretable fire segmentation masks with RGB and false-color visualizations, highlighting thermal anomalies. Performance is evaluated using IoU, Dice Score, Precision, and Recall, confirming its accuracy and flexibility across different deployment environments.
Deep dive into our solution
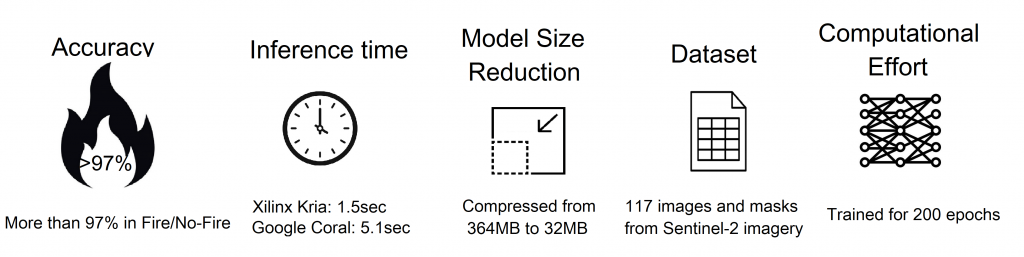
Our fire detection model is based on U-NetV2, a powerful convolutional encoder-decoder architecture widely used for semantic segmentation. It has been adapted to classify each pixel of a satellite image into two classes: fire and no fire, allowing precise localization of active fire zones. Unlike traditional models that rely solely on RGB imagery, our solution uses 10-band multispectral Sentinel-2 data, incorporating critical spectral regions such as Short-Wave Infrared (SWIR) and red-edge bands. These channels are highly sensitive to thermal signatures, burned vegetation, and subtle radiometric changes. Leveraging this hyperspectral input, the model is capable of “seeing through” cloud cover and smoke plumes, enabling accurate fire detection even under partially obscured or hazy conditions.
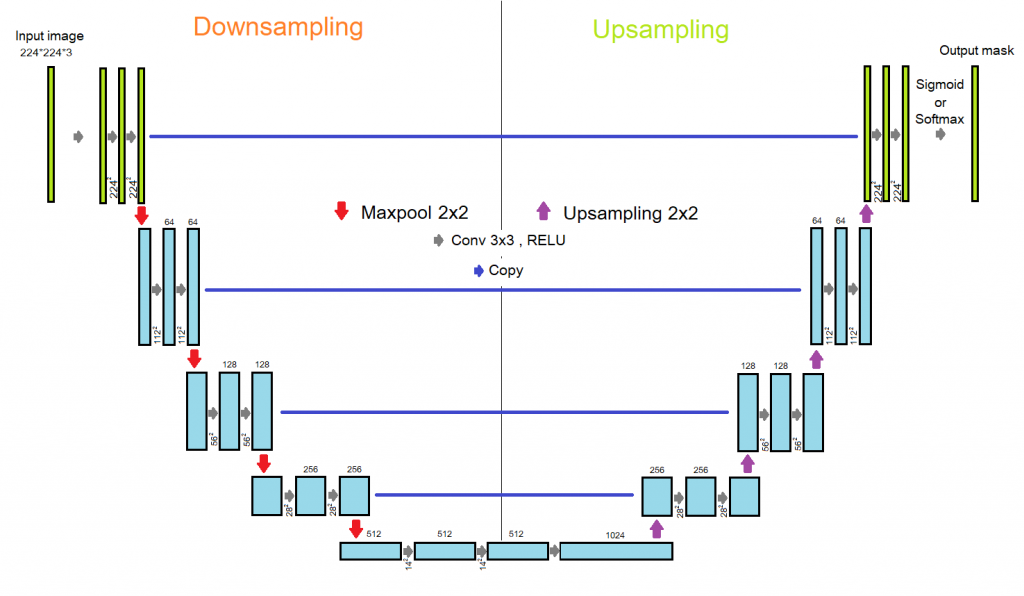
Our Vision
By extending our AI pipeline to fire detection, we reinforce our commitment to scalable, modular, and deployable AI solutions for Earth observation. This innovation not only strengthens our emergency response capabilities, but also delivers critical insights for forest monitoring, agricultural fire control, and governmental risk assessment—all from the edge, in real time, with no cloud dependency.